Tool Condition Monitoring System Using Acoustic Wave (Acoustic Emission)
- Introduction
- Solutions
- Cases
Why Acoustic Emission Technology?
During machining, problems such as tool wear, workpiece collision, and grinding wheel abnormalities (such as wear and breakage) directly affect machining quality and equipment life. Traditional monitoring methods rely on manual inspections or equipment feedback, which have problems such as slow response, low accuracy, and unpredictability.
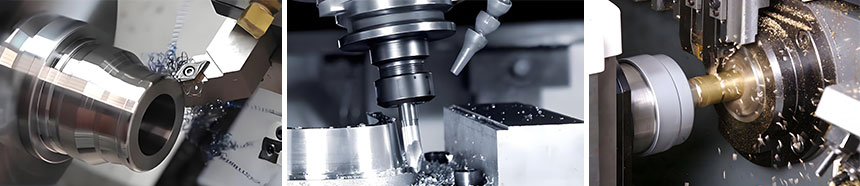
As an effective monitoring method, acoustic emission technology can capture high-frequency sound wave signals in the process of tool and workpiece processing in real time and realize millisecond-level abnormality identification. The acoustic emission monitoring system can timely detect potential problems, improve production efficiency, ensure product quality and reduce production costs by monitoring various state parameters in the processing process in real time.
Principle
During machining (cutting, grinding, punching, milling, etc.), the contact surface between the tool and the workpiece generates sound waves which are received by sensors installed on the workpiece or the tool. Different machining conditions (normal, tool wear and damage, excessive feed, workpiece finish, etc.) have different sound wave emissions. The collector analyzes the sound waves and gives the status results. The machine tool system takes measures (changing tools, stopping, reducing feed, etc.) to avoid quality accidents and extend the tool life.

Comprehensive Condition Monitoring
Various tool status monitoring (online status monitoring and automatic alarm) of wear during turning, milling, grinding, punching, drilling and other machine processes, contact position feed status, punch and drill bit damage, etc. can be done.
Monitoring Object | Key Indicator | Application Scenario |
Tool wear | Wear degree | Laths, milling machine, machining centers |
Grinding machine contact status | Contact pressure, grinding wheel loss | Grinding machines, polishing machines |
Workpiece processing quality | Surface finish, crack detection | Precision parts processing |
Equipment collision | Abnormal impact signal | CNC machine tools, standard processing equipment |
The pressure difference and the liquid temperature are one the main affecting factors of signal intensity.
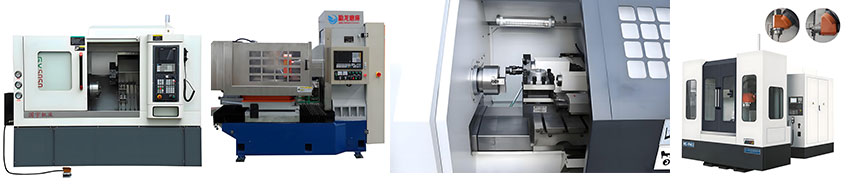
Technology Advantages
- Flexible and quick installation
- Wireless data transmission
- Auto classification and alarm
- Available for secondary development
- AI Tools available
Ultra-fast response: output shutdown signal within 1ms, quickly achieve normal contact and grinding between the grinding head and the workpiece, improve efficiency, and prevent collision damage
Strong anti-interference: digital filtering and hardware filtering are combined to eliminate environmental noise interference
High stability: IP65 protection, stable operation in -20~60℃ environment
Quick deployment: non-invasive installation, the equipment adopts magnetic suction to achieve non-invasive rapid deployment.
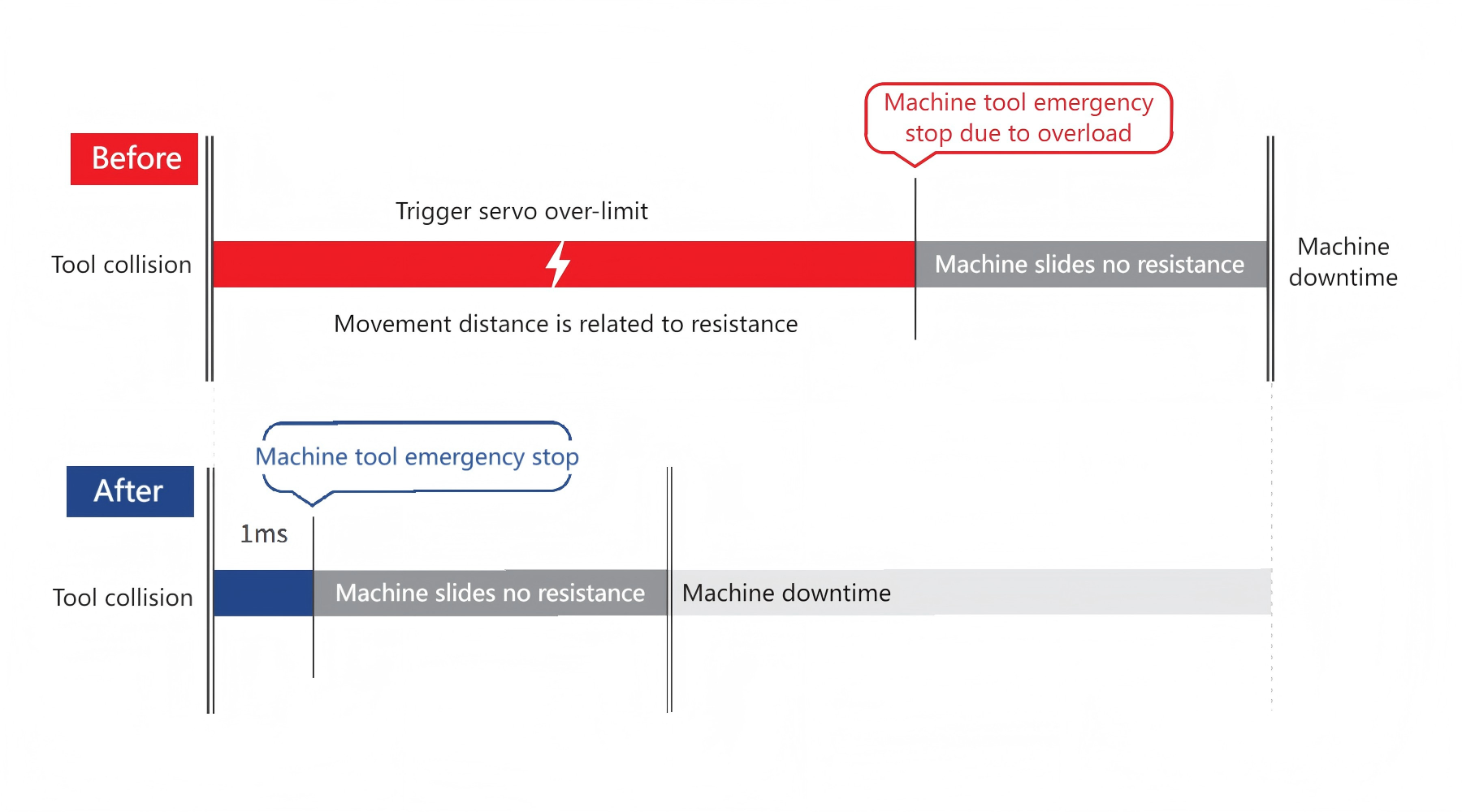
Automatic classification and alarm
Early warning mechanism: It can automatically classify the monitored status according to the thresholds and rules preset by the user. For example, the degree of tool wear can be divided into three levels: slight wear, moderate wear and severe wear. When a certain level is reached, the monitoring system will trigger the corresponding alarm, and the monitoring system will transmit the shutdown signal to the machine tool, and the machine tool will stop working.
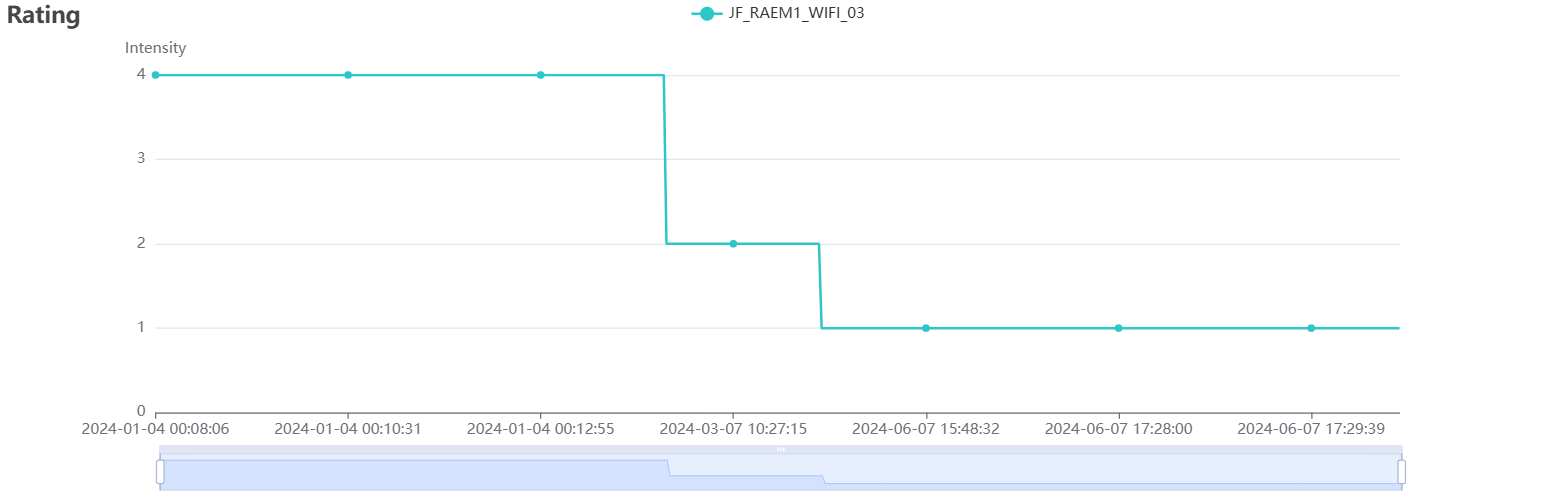
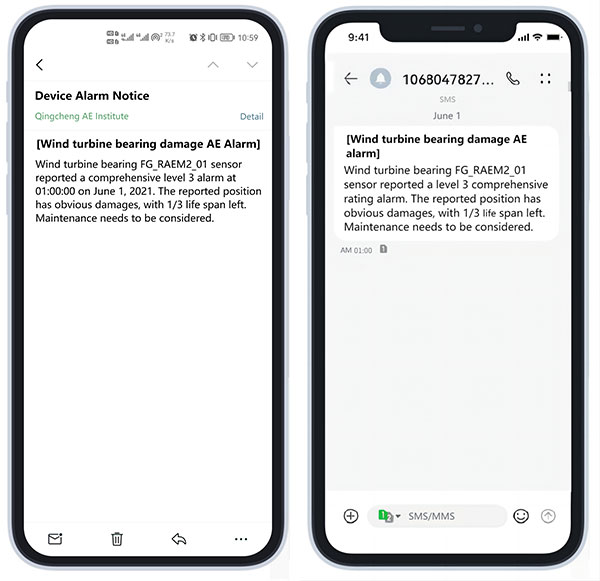
Provide secondary development interface
Provide transmission protocols and access methods, and can be seamlessly integrated into existing machine tool systems.
Communication mode: Ethernet port, RS485, WiFi, 4G, Lora;
Communication protocol: support TCP/IP, MQTT, Protobuf, user-defined protocol access to machine tool system;
Data connection: real-time transmission of acoustic emission parameters (amplitude, average signal level, RMS, etc.) and original waveform data.
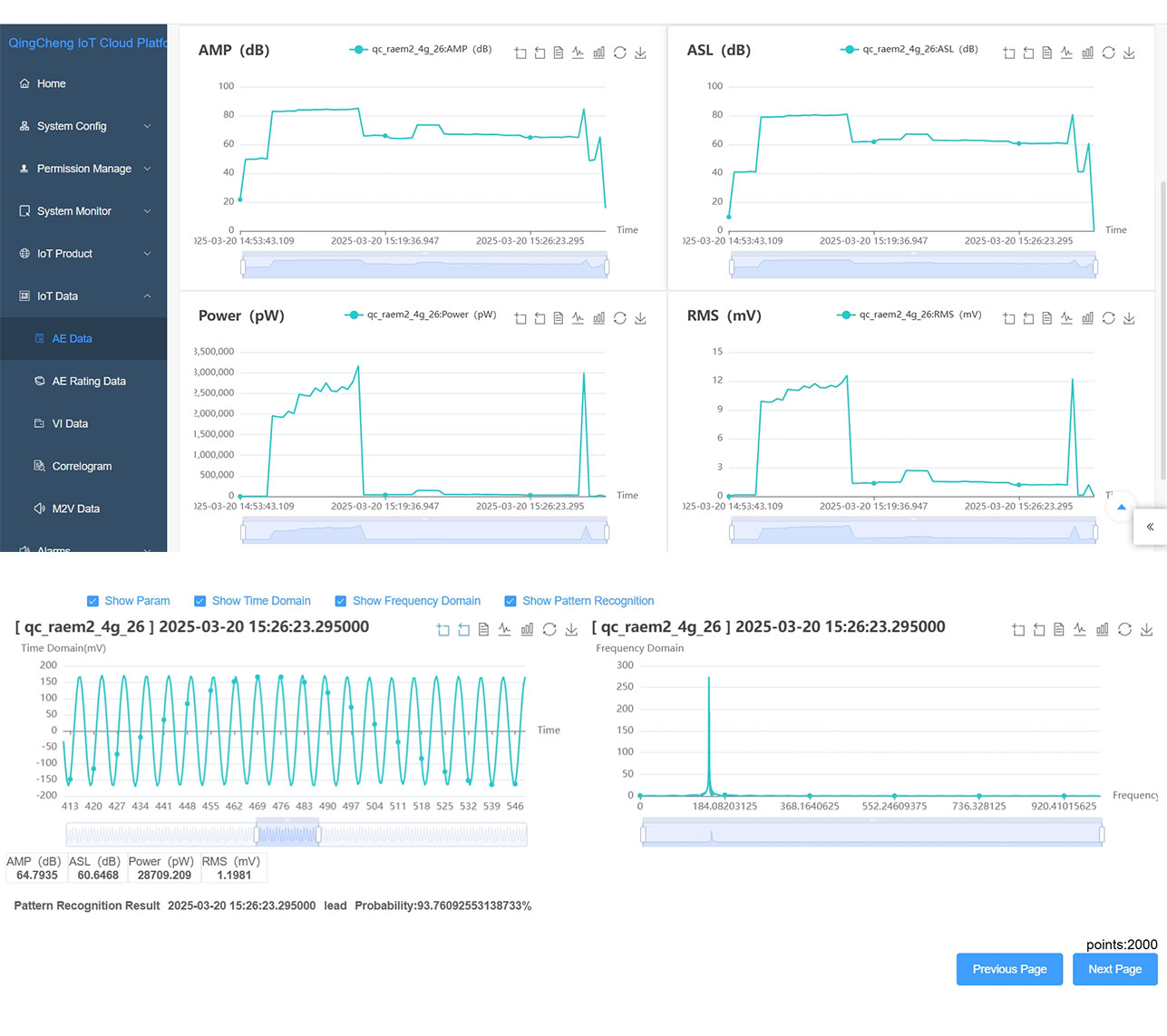
Data In-depth Analysis
SWAE analysis software provides various data views to assist in in-depth research on tool conditions such as parameter analysis, correlation graph analysis, waveform analysis, fast Fourier transform, wavelet transform, rating analysis, etc. It can customize AI autonomous learning automatic recognition and visual user interface.
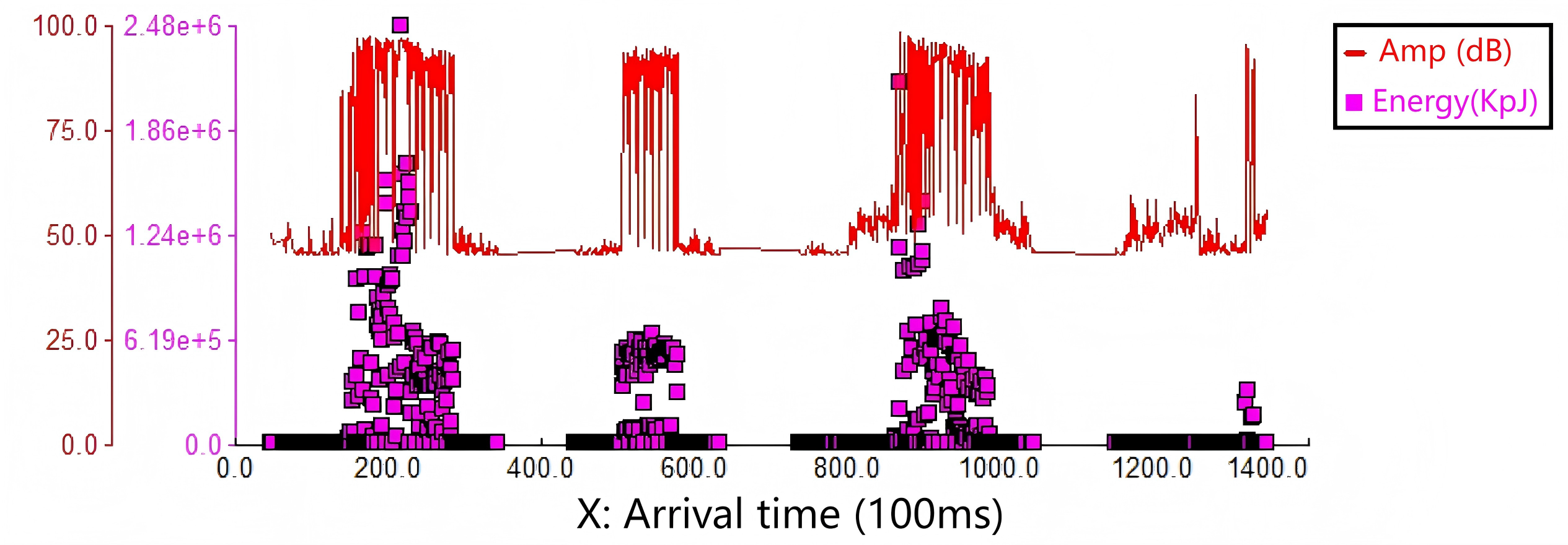
(a) Arrival time-amplitude-energy correlation diagram
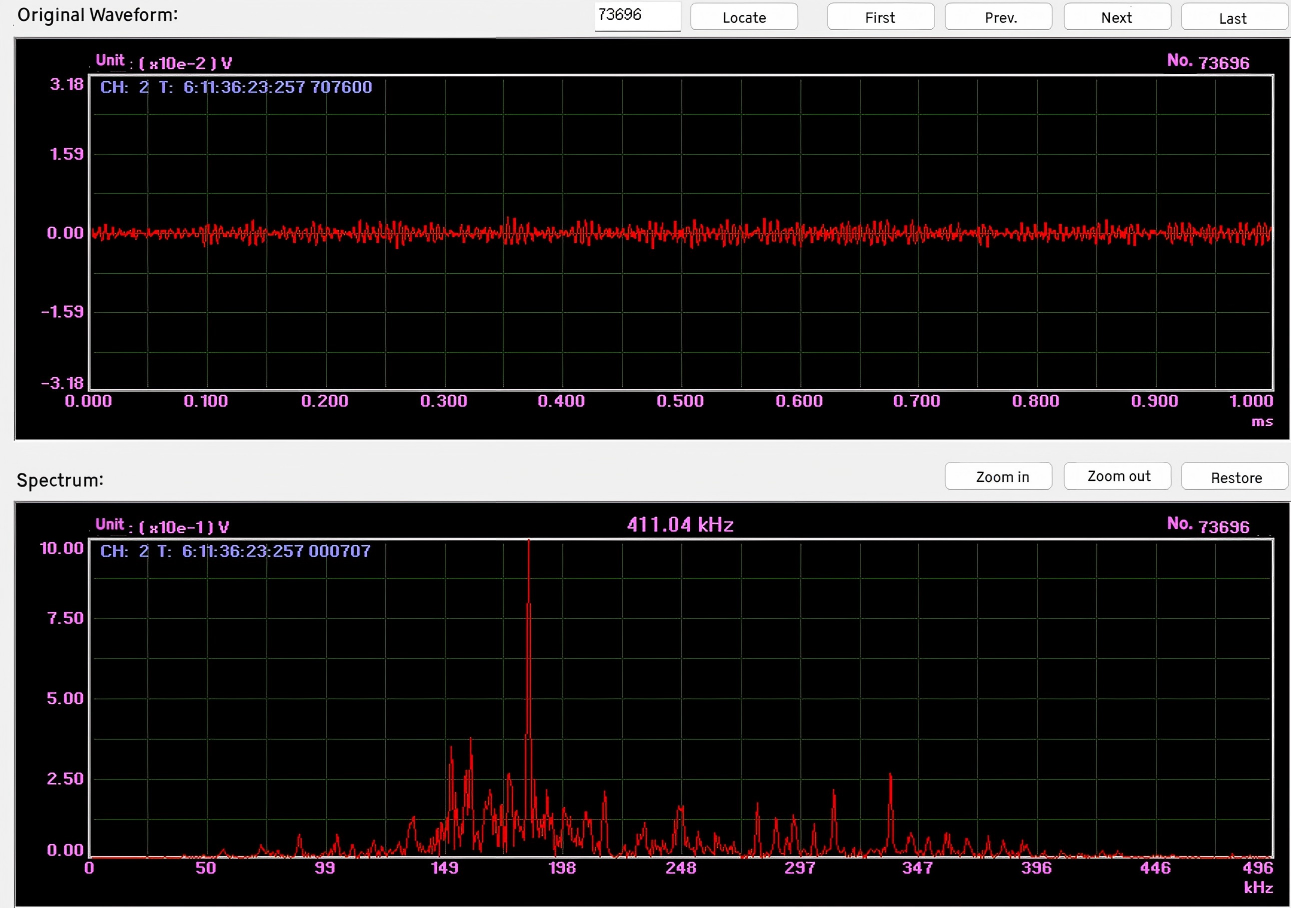
(b) Spectrum
Typical Applications
1.Tool Wear Condition Monitoring
Principle: The sound emitted during the tool machining process maps the tool wear status. The machining process emits sound wave signals, the sensor receives the sound wave signals, the collector analyzes and detects the tool wear status, and outputs the tool wear status results to the machine tool system. The system accurately determines the status and time of tool replacement, avoids quality accidents, and extends the tool use time.
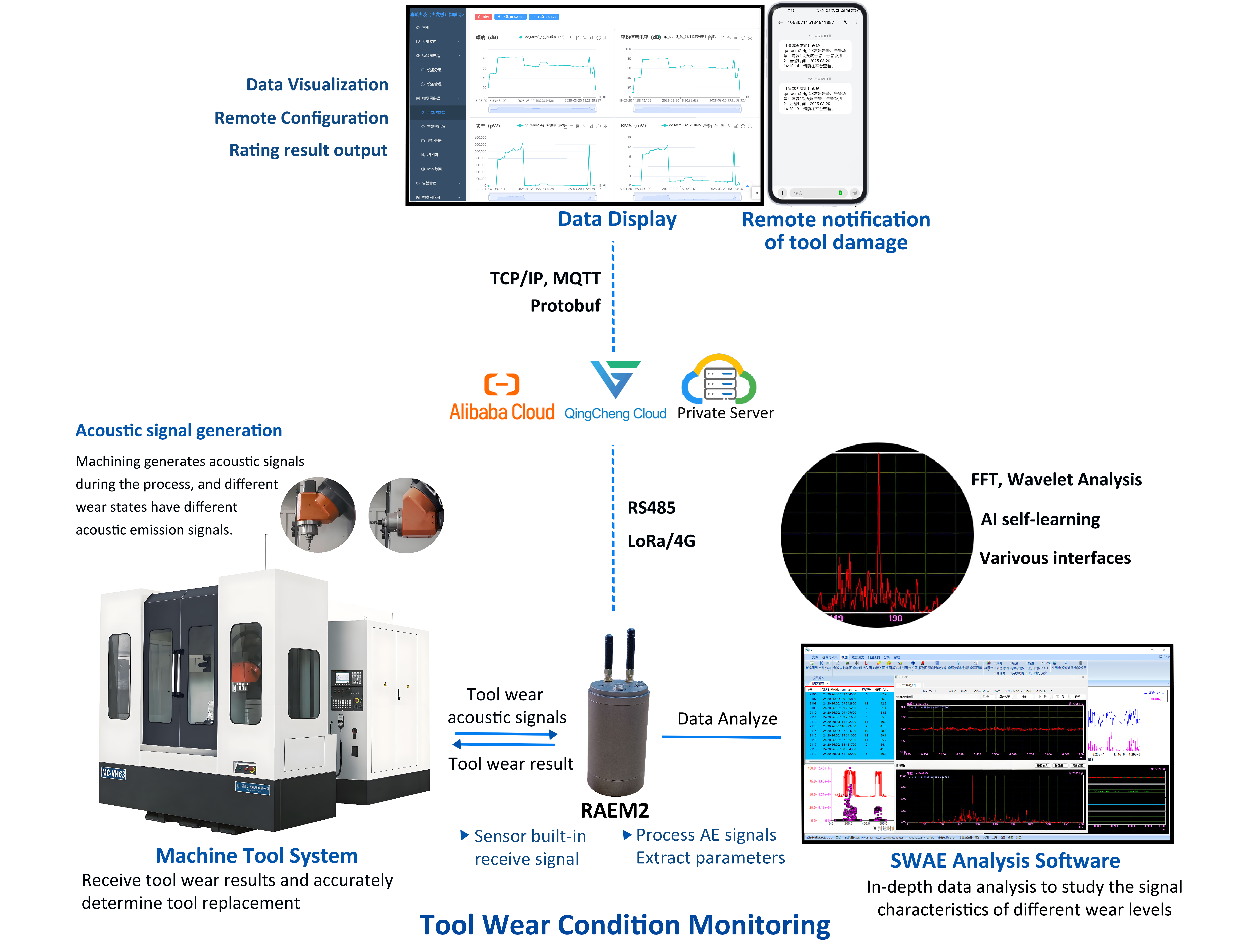
2.Automatic monitoring and control of grinding contact status
During the grinding process, the contact between the grinding wheel and the workpiece will generate an acoustic signal. The sensor receives the acoustic signal. After the RAEM1 collector processes the signal, it is uploaded to the server through the network port/WIFI/4G and other methods. Users can remotely monitor in real time.
At the same time, the RAEM1 collector determines whether the contact state is normal by analyzing signal characteristics such as amplitude, and sends a signal to the machine tool within 1ms when it is abnormal. The machine tool adjusts the grinding parameters to achieve normal contact grinding and prevent damage caused by abnormal contact or collision.
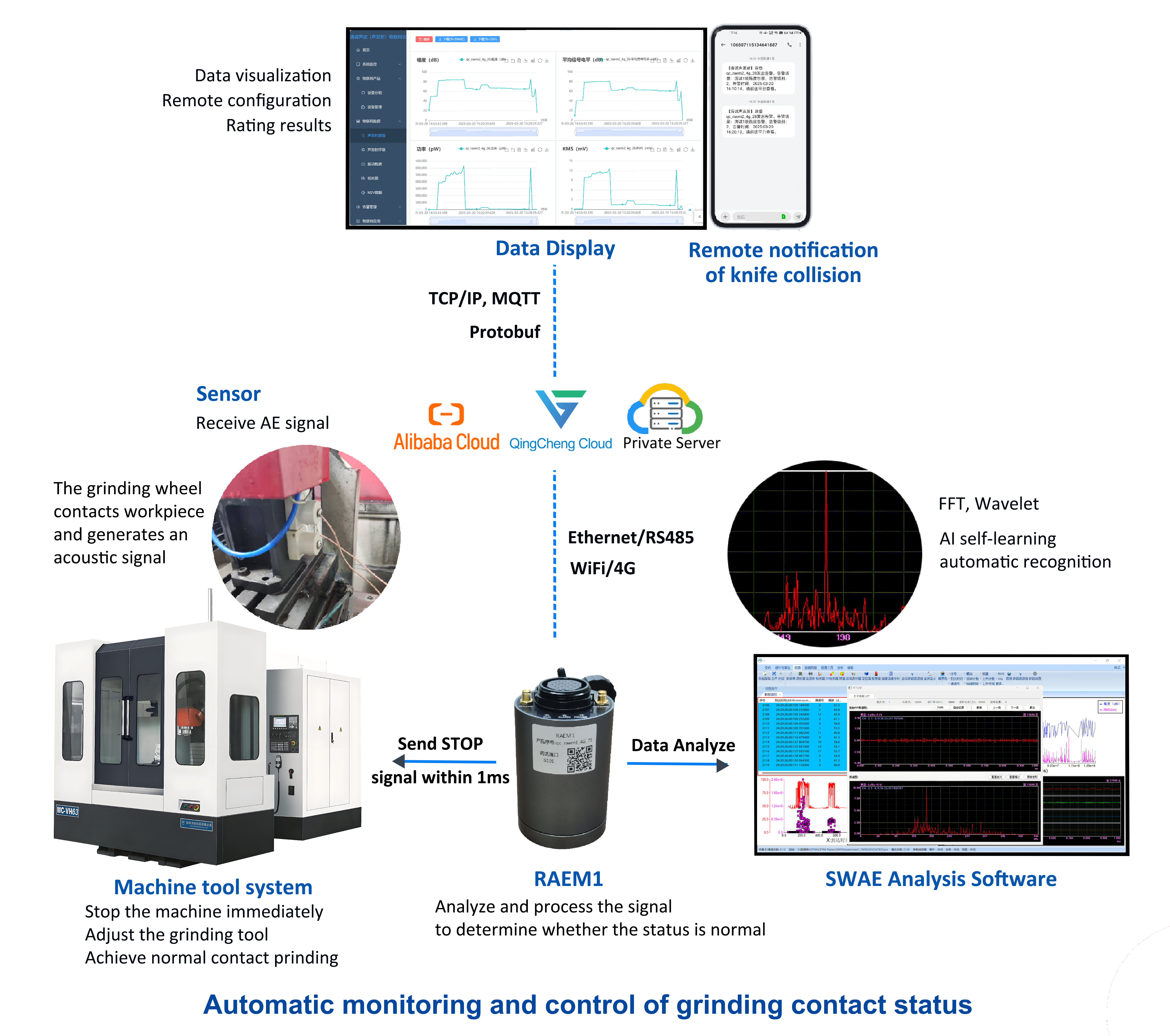
3.Grinding status monitoring
Principle: During the grinding process, the contact between the grinding wheel and the workpiece will generate an acoustic signal (reflecting the grinding state), the sensor receives the signal, the collector analyzes the signal characteristics, and the system determines whether the grinding state is normal, such as insufficient finish, damaged grinding wheel, or reaching the finish, and then takes corresponding measures.
For example, if the signal shows that the finish is not enough, continue grinding; if the grinding wheel is damaged, it will prompt to change the grinding wheel; if the finish requirement is reached, stop the machine to complete, so as to improve work efficiency, avoid quality accidents, and save grinding wheels.

4.Machine tool anti-collision system
Principle: Occasionally, machine tool failures cause tool collisions to generate acoustic signals. The sensor receives the acoustic signals and transmits them to the collector through the signal line for analysis and processing. The collector sends a collision signal to the user's machine within 1ms to control the emergency shutdown of the machine tool.
Main functions: Real-time monitoring of spindle movement and processing status, greatly reducing machine tool maintenance losses caused by equipment collision or overload, and protecting spindle safety.
Applicable to all types of machine tool programming errors, fast collisions; manual debugging, fast/slow collisions; tool/workpiece installation errors, collisions with workpieces; positioning errors, excessive cutting volume.
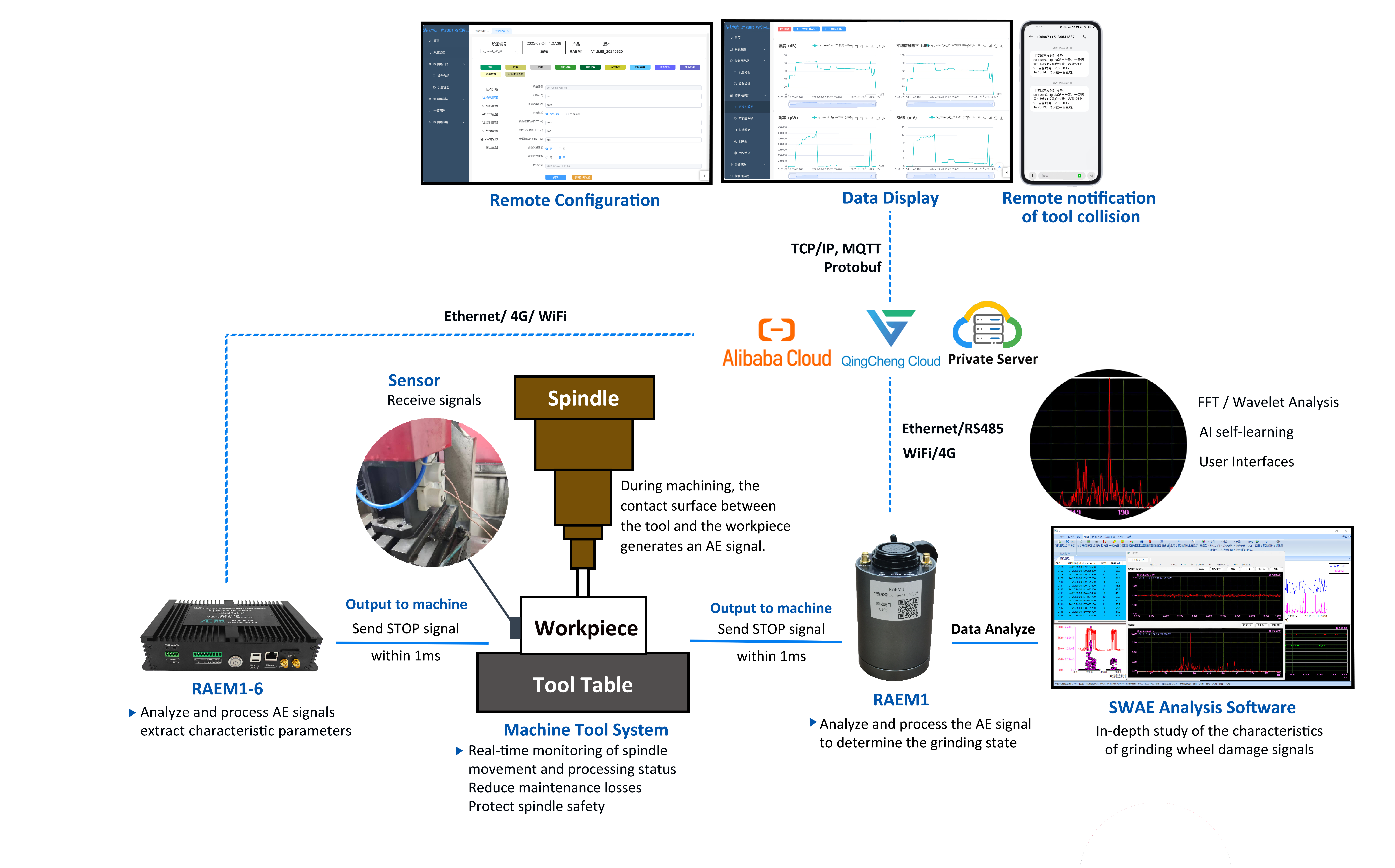
Acquisition System
1.RAEM1 - Single Channel AE System
RAEM1 is an intelligent IoT acoustic emission monitoring system that integrates automatic control signal acquisition, processing and analysis, data storage, clock synchronization and wireless communication. It has high reliability for 24-hour continuous operation, can transmit wirelessly, and is suitable for long-term continuous unattended automatic monitoring applications.
![]() |
![]() |
2.RAEM1-6 Multi-channel AE System
RAEM1-6 is a multi-channel intelligent IoT acoustic emission monitoring system that integrates automatic control signal acquisition, processing and analysis, data storage, clock synchronization and wireless communication. It has high reliability for 24-hour continuous operation and can transmit wirelessly. It is suitable for long-term continuous unattended automatic monitoring applications, such as bridge structure health monitoring, tank corrosion monitoring and other applications.
![]() |
![]() |
3.RAEM2 - Single Channel AE System with Compact Power
The RAEM2 integrated condition monitoring system integrates sensors, batteries, data acquisition modules and communication modules into a small aluminum alloy cylindrical housing (the sensor can be external). A magnet is built into the bottom of the system, which can be magnetically attracted to the surface of ferromagnetic objects. The system triggers data collection by time. RAEM2 automatically wakes up to collect data according to the sleep time. It is independent of computers and has high reliability. It is suitable for long-term continuous unattended condition monitoring. It has Bluetooth short-distance inspection function and the Internet of Things cloud platform is used for remote data monitoring and parameter configuration.
![]() |
![]() |
Hardware Technical Specifications
RAEM1 | RAEM1-6 | |
Channel | Single channel or networking to a multi-channel system | 2 to 6 channels per case; Cascade use |
Acquisition Method | Threshold/ time trigger | Threshold/ time trigger |
Sampling Rate | 2MSP/s; 16-bit | Single channel up to 2MSP/s; 16-bit |
System Noise / Dynamic Range | ≤30dB / 70dB | ≤30dB / 70dB |
Input Bandwidth | 10kHz-800kHz | 10kHz-800kHz |
Analog Filter | 30kHz, 125kHz two high-pass filters, 80kHz, 175kHz two low-pass filters, default 30kHz~80kHz, 125kHz~175kHz two band-pass filters, factory fixed | 30kHz, 125kHz two high-pass filters, 80kHz, 175kHz two low-pass filters, default 30kHz~80kHz, 125kHz~175kHz two band-pass filters, factory fixed |
Digital Filter | 256-order FIR filter, any value setting of pass-through, high-pass, low-pass, band-pass in the frequency range of 0kHz~1000kHz | Any value can be set in the frequency range of 0kHz~1000kHz, such as direct pass, high pass, low pass, band pass (used in conjunction with analog filter) |
Sensor | Built-in preamplifier series sensors, three types of built-in preamplifiers are available: 28V40dB, 12V34dB, 5V26dB | Built-in preamplifier series sensors, three types of built-in preamplifiers are available: 28V40dB, 12V34dB, 5V26dB |
Data Output | Waveform, parameters, parameter rating | Waveform, parameters, parameter rating |
AE Features Parameters | Arrival time, amplitude, counts, energy, rise time, duration, RMS, ASL, peak frequency, centroid frequency, 5 partial power spectrum segments | Arrival time, amplitude, counts, energy, rise time, duration, RMS, ASL, peak frequency, centroid frequency, 5 partial power spectrum segments |
Memory Capacity | 64G (expandable to 512G) | 64G (expandable to 512G) |
Clock Synchronization | / | Can serial port (wired) cascade large channels, 36 channels synchronous clock accuracy ≤ 10us |
Communication Method | 4G, Ethernet, WiFi, RS485 | 4G, Ethernet, WiFi |
Work Temperature | -20℃~60℃. (WiFi version is 0℃~60℃) | LAN: -20~60℃; WiFi: 0℃~60℃ |
Power Supply | 12VDC | 12VDC |
Dimension | Cylinder diameter φ62mm, height 100mm | Length × width × height: 23.2cm × 12.5cm × 5.2cm |
Weight | 220g | 1.6kg |
RAEM2 Technical Specifications | |||
Channel | Single channel | 4G supported frequency bands |
LTE-FDD:B1/B3/B5/B8 LTE-TDD:B34/B38/B39/B40/B41 |
Acquisition Method | Continuous acquisition mode, interval acquisition mode, timing acquisition mode (Note: Lora version only has interval acquisition mode) | Bluetooth communication distance range | The maximum distance in the unobstructed area is 13.0m |
Sampling Rate | 2MSPs; 16bit | Smartphone Inspection | Bluetooth |
System Noise/ Dynamic Range |
Internal sensor version: ≤40dB/60dB; External sensor version: ≤30dB/70dB |
Power Supply |
Multiple options available: 1. External 8.4VDC power supply 2. Built-in rechargeable battery (3000mAh@8.4V) 3. Built-in low self-discharge rate lithium-ion battery (7000mAh@8.4V, non-rechargeable) |
Input Bandwidth | 10KHz-400KHz | Charging Voltage | 8.4V |
Digital Filter | 128 steps, 0-1000kHz adjustable (filter range is related to sampling rate, the maximum is 1/2 of sampling rate) | Sampling length |
2000KSPs: 500~15000µs 1000KSPs: 1000~30000µs 500KSPs: 2000~60000µs 200KSPs: 5000~150000µs |
Sensor | 150KHz(60KHz-400KHz)/40KHz(15KHz-70kHz)可选 | Trigger | Time trigger |
Data Output | Parameters (amplitude, RMS, power, ASL), waveform (Note: Lora version does not support sending waveform data) | Battery life in timed acquisition mode |
The max theory working time (when waking up once a day for 1 second): 3 years (7000mAh lithium-ion battery), or 1 year (3000mAh rechargeable battery) |
Communication | 4G/LoRa, RS485 | Sleep time accuracy | ±1min (note: LoRa version: 3min) |
Work Temperature | -20℃~60℃. (WiFi version is 0℃~60℃) | Battery life in continuous mode |
15h (using rechargeable battery); 24h (using lithium-ion battery) |
Dimension |
Diameter φ60mm, height 105mm (including 150k sensor, excluding antenna); height 117mm (including 40k sensor, excluding antenna) External sensor: diameter φ60mm, height 105mm (excluding antenna) |
||
Weight | <500g (including battery, magnet, antenna) |
Sensor
GI150/1 | W800/1 | GI40 | W500/1 | |
![]() |
![]() |
![]() |
![]() |
|
Resonant Frequency | 150KHz | 600KHz | 40KHz | 500KHz |
Frequency Range | 60KHz~400KHz | 50KHz~800KHz | 15KHz~70KHz | 100KHz~1000KHz |
Sensitivity | >75dB | >65dB | >75dB | >65dB |
Preamp | 40dB28V | / | 40dB28V | / |
Connector | SMA | BNC | BNC | BNC |
IP Degree | IP62 | IP68 | IP62 | IP68 |
Temperature | -20℃~50℃ | -20℃~120℃ | -20℃~50℃ | -20℃~120℃ |
Dimension(mm) | Φ22×25.5 | Ф19×15 | Ф30×57 | Ф19×15 |
Features | Built-in preamp | Waterproof broadband | Built-in preamp | Waterproof broadband |
* If the sensor has built-in preamp or waterproof requirement, please contact us for details.
Cloud Platform Introduction
Qingcheng IoT cloud platform, Alibaba Cloud platform, Amazon Cloud platform, customer's private cloud platform, etc. Support TCP/IP, MQTT, Protobuf, and user-defined protocols to access customer's private cloud platform, and the cloud platform can be privately deployed to the user's local server.
Qingcheng IoT Cloud
Qingcheng Cloud Platform is a data management cloud platform that integrates data display, data query, remote configuration, intelligent monitoring, and alarm push. It has the functions of real-time/historical data analysis and viewing, remote configuration parameters, automatic rating, and alarm notifications.
-
Remote configuration:including parameter configurations (such as sampling rate, sampling length, sampling times, sampling interval), filter configuration, timing configuration, etc., remote firmware upgrade, eliminating the need for manual on-site maintenance.
- Remotely view real-time/historical data: View monitoring data in real time and query historical data for any time period.
-
AE characteristic parameters: amplitude, ASL, energy, duration, ring count, rise time, RMS, rise count, peak frequency, centroid frequency, and 5 partial power spectrum segments.
Note: Data can be uploaded to the user-specified private cloud platform according to user requirements.
- Rating function: The acoustic emission data adopts an automatic grading algorithm, and the intensity level is obtained from the acoustic emission impact parameters. The rating results can be viewed remotely on the cloud
-
Rating parameters: amplitude, ASL, energy, duration, ring count, rise time, RMS, rise count
-
Correlation diagram: Use the two acoustic emission parameters contained in the parameter table as the horizontal and vertical coordinates to draw a correlation curve or distribution point diagram, line diagram, etc.
-
Alarm notification: When an abnormality is found, the alarm information is immediately sent to the user. The alarm information is automatically pushed through email and SMS. Alarm mechanism process: sensor collects sound wave signals → collector analyzes and processes signals → cloud platform → alarm push.
-
Data download: The data format can be CSV format or our parameter file or waveform file (.PRA and .AED) format.
Application Cases
Case 1: Tool Collision Acoustic Emission Monitoring
In March 2024, our company was invited by a machinery company to conduct a tool collision experiment on a machine tool.
The machine spindle of the grinder was fixed on the left, and the grinder rotated on the spindle. The object to be polished (tool) was installed on the right bracket. Because the spindle was rotating, there was a lot of noise. If the sensor was installed on the fixed bracket behind the spindle, there would be multiple structural parts in the middle, and the attenuation was very large. Since the bracket would rotate to adjust the angle to polish both sides of the device (the rotation angle was about 150°), the acoustic emission sensor was installed on the right bracket (i.e. the back of the tool position).
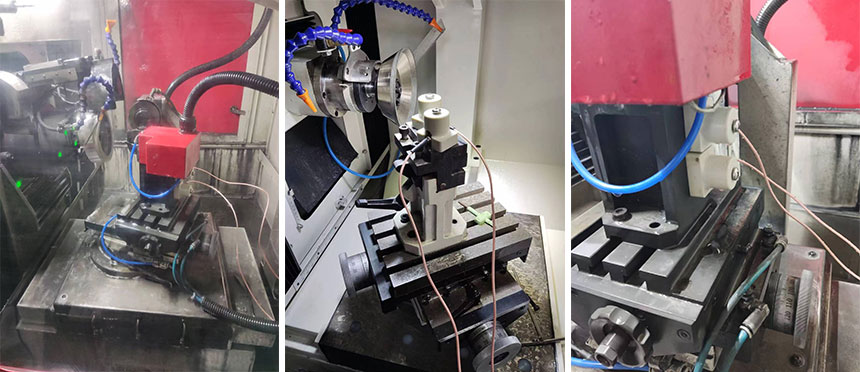
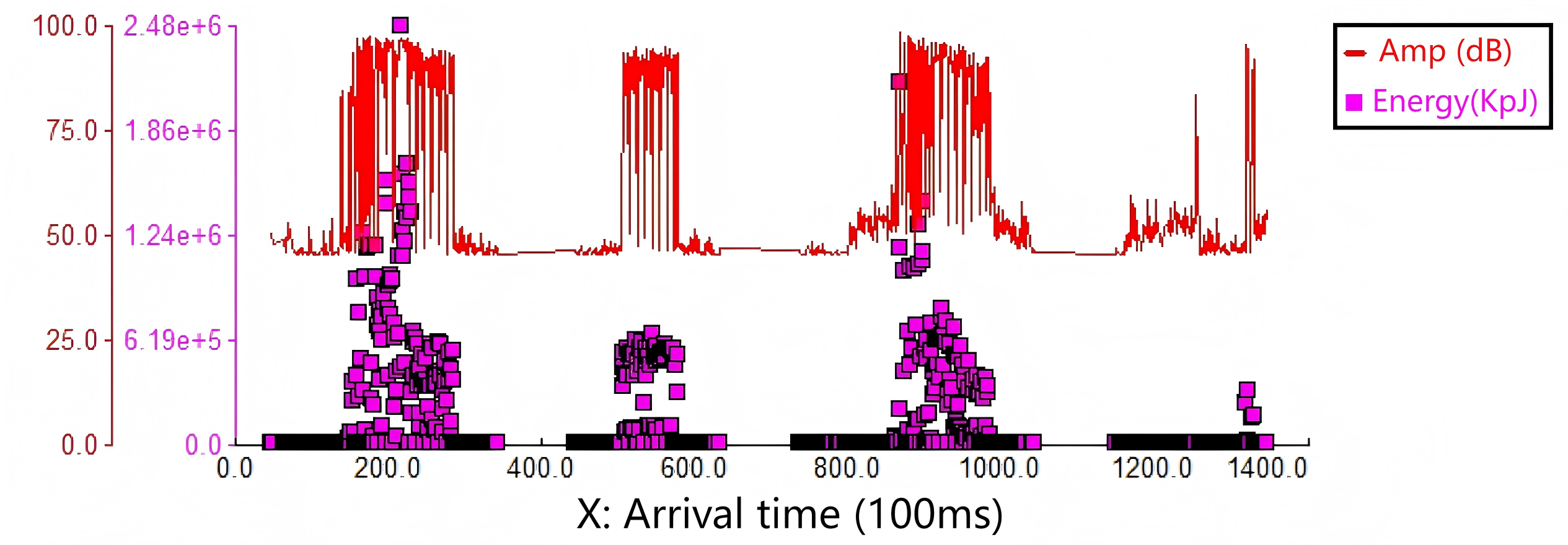
Case 2: Acoustic Emission Monitoring of Machining Tool Wear
The acquisition module was placed on the tool, and the collected data was uploaded to the cloud. A certain algorithm was used to determine whether there was a fault or wear, and an alarm message was pushed to the client. This avoided tool failure and equipment damage, reduced downtime, and improved production efficiency.
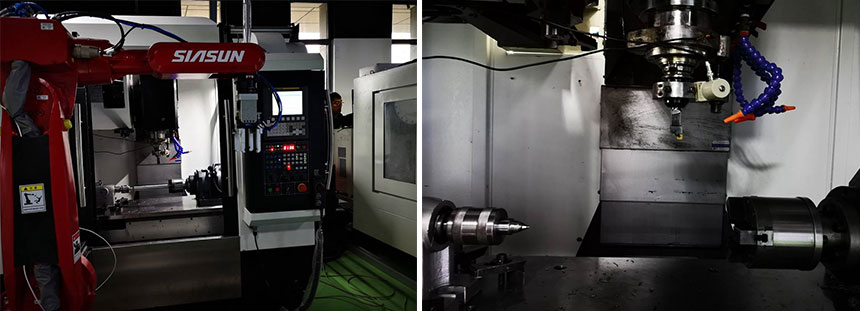

Signal counts/energy-arrival time diagram
Key points:
1. The use of appropriate sensors and filters can effectively eliminate on-site noise interference. The main acoustic emission signals generated during the processing are workpiece material cracking and tool-workpiece friction signals;
2. Because the original data covers multiple types of acoustic emission signals, it is not easy to distinguish a single acoustic emission characteristic parameter before and after comparison;
3. Combining multiple acoustic emission characteristic parameter comparisons, through combined identification such as energy and ringing counts comprehensive judgment method, the early signal of tool wear can be effectively identified in advance.
Contact Form
Please complete the form below to request your quote. We will be in contact as soon as possible.